Artificial Intelligence and Data Extraction (Part 1 of 3)
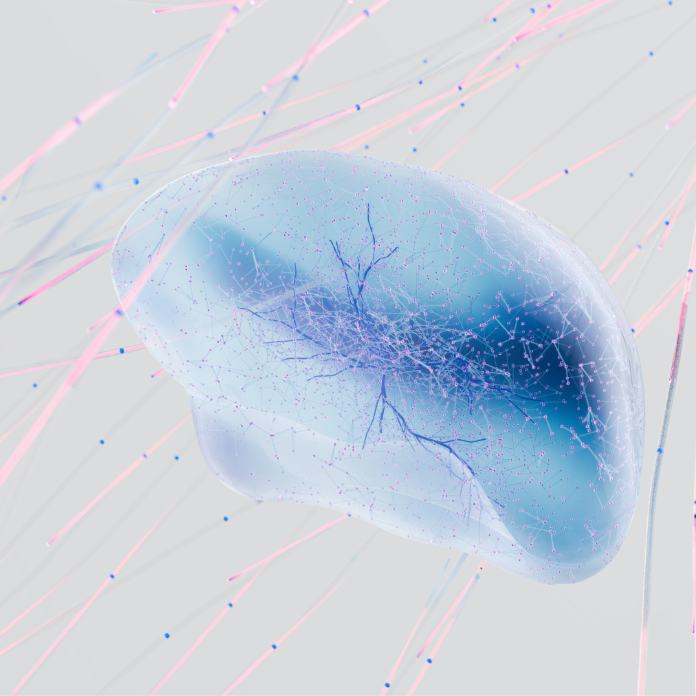
Data extraction from documents is a critical task for businesses across various industries. From invoices and receipts to legal contracts and medical records, companies need to extract information from large volumes of unstructured data in order to gain insights, reduce costs, and improve efficiency. However, manual data extraction is a time-consuming and error-prone process that can lead to inefficiencies, delays, and inaccurate data. This is where #AI comes in, offering a solution to automate the data extraction process and enable businesses to make more informed decisions based on accurate, structured data.
Understanding the relationship between #DataExtraction and #ArtificialIntelligence entails exploring the various challenges and opportunities that are presented by technology to businesses today. We need to understand the motivations behind the push for automation and the potential pitfalls of making the wrong decisions in the choice of technology solutions.
Audience
This blog series may be useful for both business leaders who need to make strategic decisions regarding automation and also technology teams who need to support the business functions with the implementation and engineering support for these initiatives.
In this first part of our exploration, we will look at business motivations that drive organizations towards automation projects and the technology challenges that stand in their way. Clarity on these two fronts may help get started with this journey in a more focused manner.
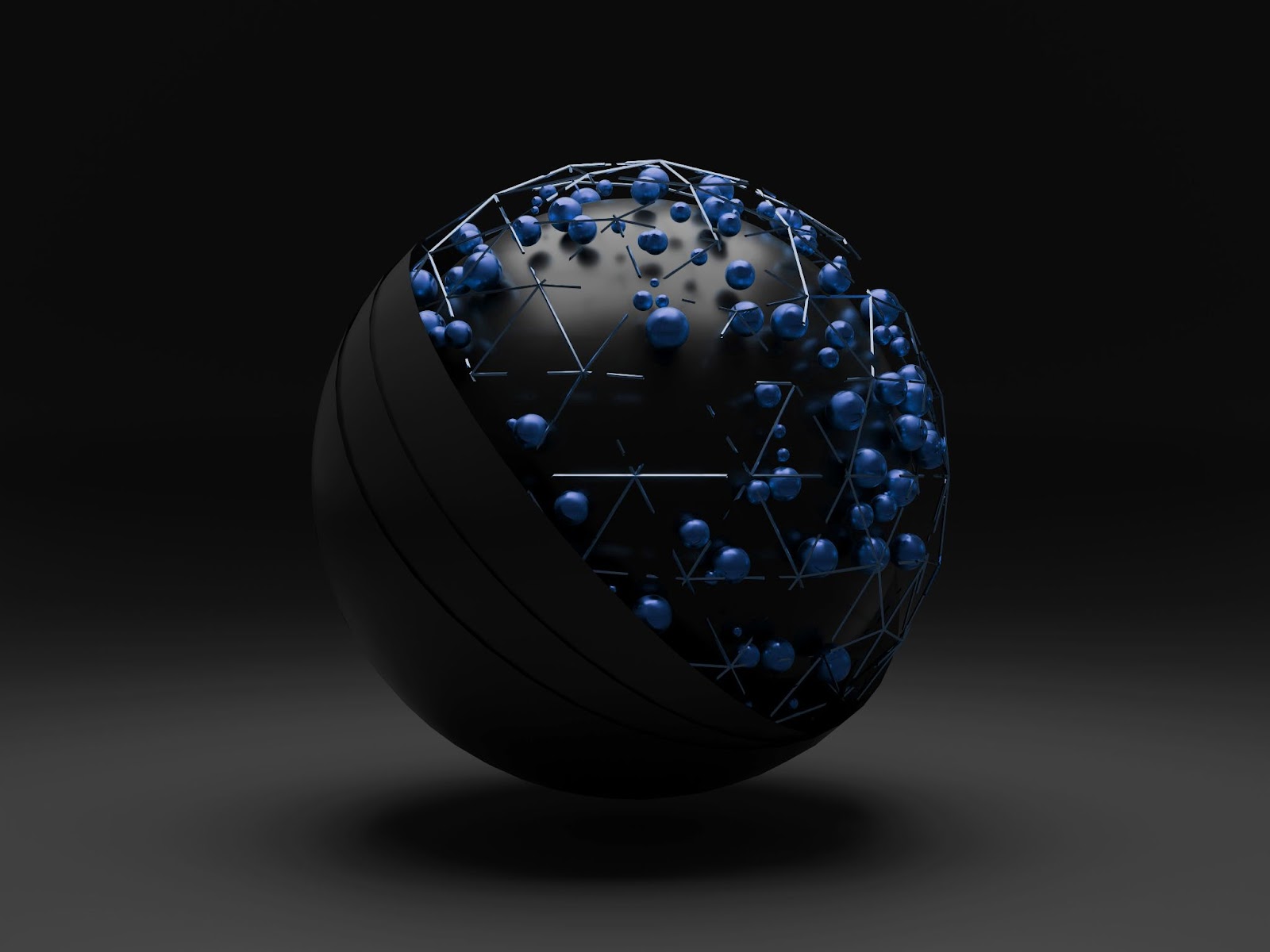
Business Motivations
One of the biggest challenges businesses face when it comes to data extraction is the sheer volume of unstructured data. Companies need to extract valuable information from a wide range of sources, including emails, PDFs, and images, which can be a daunting task. This requires significant manual effort, leading to delays and inaccuracies, which can have a negative impact on business performance. Furthermore, businesses need to ensure that the extracted data is accurate and reliable, which can be difficult to achieve with manual data extraction.
There are several goals that businesses aim to achieve with their automation projects:
- Increased Efficiency: Businesses want to extract data from documents faster and more accurately than manual processes allow. By eliminating manual data entry and repetitive tasks, businesses aim to achieve higher productivity and process documents more efficiently. With automation, businesses can extract data from multiple documents, and document types, simultaneously, accelerating decision-making processes and improving overall operational efficiency.
- Cost Reduction: Automating document data extraction can lead to cost savings by reducing the need for large volumes of manual labor. Businesses can allocate resources more effectively, streamline operations, and minimize errors, ultimately reducing operational costs.
- Improved Accuracy: Manual data entry is prone to errors, such as typos or misinterpretations. Automation improves accuracy by leveraging machine learning algorithms and optical character recognition (OCR) technology, resulting in more reliable and error-free data extraction.
- Enhanced Scalability: As businesses grow and document volumes increase, manual data extraction processes can become overwhelming and time-consuming. Automation allows for scalability, enabling businesses to handle larger volumes of documents without sacrificing accuracy or speed.
- Faster Technology Adoption: Often businesses that have a degree of manual document perusal in their processes are at a disadvantage in the adoption of new technologies. Many opportunities to evolve technologically may be lost simply because existing products do not mesh well with manual steps or the extended timelines of the manual stages. Bringing an end to end automation workflow removes these limitations and gives control back to the technology teams.
- Standardization and Consistency: Automation ensures consistent data extraction by following predefined rules and formats. This standardization reduces variations and discrepancies in extracted data, enhancing data quality and enabling better analysis and decision-making.
- Integration with Existing Systems: Automated document data extraction processes can be seamlessly integrated with existing business systems, such as customer relationship management (CRM) software or enterprise resource planning (ERP) systems. This integration facilitates data flow across different departments and improves overall data management.
- Compliance and Regulatory Requirements: Automation helps businesses meet compliance and regulatory requirements by accurately capturing and managing data. It ensures adherence to data privacy laws, audit trails, and data security standards, reducing the risk of non-compliance.
- Improved Customer Experience: By automating document data extraction, businesses can process customer requests and enquiries more efficiently. This leads to faster response times, reduced customer wait times, and an overall improved customer experience.
- Strategic Insights and Decision-making: Automated data extraction processes provide businesses with access to accurate and structured data. This data can be further analyzed, leading to actionable insights, informed decision-making, and the ability to identify trends, patterns, and opportunities for business growth.
It's important to note that businesses may have additional specific goals depending on their industry, processes, and unique requirements when automating document data extraction. These will impose additional constraints or steps into the overall process further emphasizing the value automation will bring to these processes.

Technology Challenges
There are several technology challenges when it comes to using AI for data extraction. One of the key challenges is training the AI models to accurately extract data from various document types. This requires a large amount of training data, which can be difficult to obtain, and can also be time-consuming and expensive. Additionally, the AI models need to be updated regularly to ensure that they remain accurate and effective in extracting data.
Here are some common challenges that technology solutions for data extraction pose:
- Document Variability: Documents can come in various formats, layouts, and structures. AI systems need to be trained to handle different document types and variations effectively. Dealing with unstructured or semi-structured data, handwritten text, or documents with complex tables and diagrams can pose challenges for accurate data extraction.
- Data Quality and Accuracy: AI models heavily rely on the quality and accuracy of training data. If the training data contains errors or biases, it can impact the performance of the AI system. Ensuring high-quality training data and minimizing biases are essential for achieving accurate data extraction results.
- Limited Availability of Labelled Data: Training AI models for document data extraction often requires large amounts of labelled data. However, labelled data can be scarce or costly to obtain, especially for specialized or domain-specific document types. Acquiring and annotating sufficient training data can be a significant challenge.
- Extraction of Contextual Information: Extracting data from documents often requires understanding the context and meaning of the information. AI systems may struggle to interpret complex or ambiguous language, especially in legal documents, contracts, or technical papers. Capturing and processing contextual information accurately can be a challenging task.
- Handling Multilingual Documents: Documents may be written in different languages, requiring AI systems to support multilingual capabilities. Overcoming language barriers, accurately recognizing and extracting text in different languages, and handling language-specific nuances pose challenges for AI-based data extraction.
- Adaptability to Changing Document Formats: Businesses frequently encounter new document formats, templates, or versions. AI systems need to be flexible and adaptable to accommodate changes in document structures and formats. Regular updates and retraining may be required to ensure optimal performance and compatibility.
- Handling Noisy or Incomplete Data: Documents may contain errors, missing information, or inconsistencies that can affect data extraction accuracy. Noise in the data, such as smudges, poor image quality, or scanned documents, can make text recognition and data extraction more challenging. AI systems need to be robust enough to handle noisy or incomplete data effectively.
- Privacy and Security Concerns: Document data often contains sensitive or confidential information. Ensuring data privacy and security during the data extraction process is crucial. AI systems should incorporate appropriate measures to protect data confidentiality, comply with regulations, and prevent unauthorized access or data breaches.
- System Integration and Compatibility: Integrating AI-powered data extraction systems with existing IT infrastructure and workflows can be complex. Ensuring compatibility, seamless data flow, and interoperability with other systems or databases may require additional development and customization efforts.
- Explainability and Transparency: AI models used for data extraction often operate as black boxes, making it challenging to explain or interpret their decisions. Businesses may need to address the explainability and transparency of AI systems, especially in regulated industries or when human oversight is required.
Overcoming these challenges requires continuous research and development, leveraging advanced AI techniques, and considering specific domain knowledge and requirements.
In the next section we will look at various AI product options that are available for use and some of the pre-requisites the businesses have to put together before diving in.